By Kofikuma Dzotsi
When you look at a CIBO parcel report, you see a graph showing simulated yields for that parcel across several years (Figure 1). If you select another parcel, you will most likely see different yield values and different year-to-year patterns. This is true for each parcel across the geography covered by CIBO’s platform.
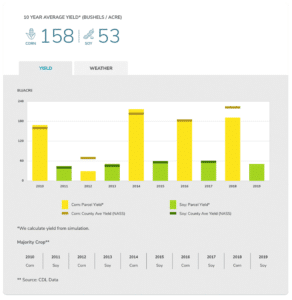
These simulated yields can help growers compare the productivities of different parcels over time, and the potential benefits of this technology are vast. But it leads to one question: How does CIBO calculate these yields for each parcel across the corn belt — and beyond? The answer lies in the core technology used by CIBO to model crops in virtually any environment.
If you wish to know crop yield in a given year on a given parcel in Iowa, for example, one possibility is to physically travel to that parcel and conduct a field experiment. In this scenario, you’d grow a crop, then measure the yield when the crop matures. This process would undoubtedly provide the desired answers; however, it cannot be repeated for all parcels across the U.S. — and for all years of interest — because such experiments would simply be too expensive and impossible to manage.
But what if we constructed a digital model-plant, gave it the main characteristics of a real plant (roots, leaves, flowers, grains, etc.), then asked the computer to “grow” the plant by essentially mimicking the physiological behavior of a real plant? Once we have a model-plant that grows on a given parcel in a given year, it becomes possible to expand this process to other parcels, other years, and other crops. This is exactly the process CIBO’s crop simulation engine uses to predict crop yield.
The Four Factors of Crop Yield
The first step in building this kind of crop simulation engine is identifying the components that will go into the model-plant. Remember: the model-plant is an abstract, simplified representation of a real plant‚ not a physical model. Therefore, we need to select the components that are most influential to crop yield to keep the model manageable. The main components that are important determinants of crop yield are:
- soil properties (soil pH, soil texture, soil organic matter, etc.),
- weather (temperature, radiation, precipitation),
- management (planting date, planting density, amount of nitrogen applied during the season, etc.), and
- the characteristics of the specific crop variety grown (in the case of corn, relative maturity and the number of leaves the plant produces).
Once these components are identified, the next step involves defining the mathematical relationships linking them together. For example, when a corn plant grows in the field, the time it takes the crop to mature strongly depends on the temperature of its growing environment. So, the time to maturity would be calculated using some function of temperature.
As you can imagine, building all these relationships is a major undertaking — this step involves an interdisciplinary collaboration among many scientists who contribute knowledge from several disciplines about how the plant interacts with its environment. At this point we have constructed a mathematical model of the plant, which is basically a quantitative version of the model-plant.
Real Math Finds the Simulated Yield
We can think of this mathematical model as a large system of equations linking the plant characteristics to environmental variables and management practices (Fig. 2). Like all systems of equations, selecting appropriate initial conditions and solving them will provide us with useful solutions. In our case, the system of equations is very complex, so we use some sophisticated techniques to solve it. The model is then written in a language that computers can understand. Running the model is equivalent to solving the system of equations using a computer to obtain meaningful crop outputs, which is essentially the same as simulation. Among many other variables, our solution of interest is crop yield.
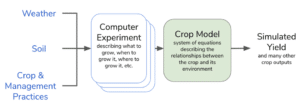
So, the next time you see CIBO-simulated yield (or productivity) for a parcel, remember that a complex simulation process underpins that one yield value, from a model-plant all the way to solving a massive mathematical model– and it can all be done from a computer rather than a field in Iowa.
About Kofikuma Dzotsi
Kofikuma Dzotsi is a Principal Crop Scientist at CIBO Technologies, a science-driven software startup. Prior to CIBO, he worked on developing and applying crop models in the public and private sectors. He holds an MS and Ph.D. in agricultural and biological engineering from the University of Florida.
Continue Learning About Regenerative Agriculture
Return to the pathway to build your knowledge of regenerative agriculture
Return to Pathway